Introduction to LLM Knowledge Graph Drug Purpose
In recent years, the field of drug discovery has experienced a paradigm shift due to advancements in artificial intelligence (AI) and data science. A significant innovation at the forefront of this revolution is the LM knowledge graph drug purpose framework. Combining the power of large language models (LMs) with knowledge graphs, this approach is transforming how drugs are developed, analyzed, and repurposed. This article delves into the intricate relationship between these technologies and their potential to accelerate breakthroughs in medicine.
Table of Contents
Understanding the Basics of LLM Knowledge Graph Drug Purpose
To fully appreciate the impact of LLM knowledge graph drug purpose, it is essential to break down the core components:
- Large Language Models (LLMs): These AI systems are designed to understand and generate human-like text based on vast datasets. They excel in natural language processing tasks, including summarization, translation, and contextual understanding of complex topics.
- Knowledge Graphs: These are structured networks of data points representing relationships between entities. In the pharmaceutical domain, knowledge graphs organize information about drugs, diseases, genes, and biochemical pathways.
When combined, LLM knowledge graph drug purpose integrates the linguistic capabilities of LLMs with the relational insights of knowledge graphs to identify novel drug applications, improve precision medicine, and enhance the efficiency of research pipelines.
How LLM Knowledge Graph Drug Purpose Works
The synergy between LLMs and knowledge graphs operates through several key mechanisms:
- Data Integration: Knowledge graphs aggregate data from diverse sources, including scientific literature, clinical trials, and genomic databases. LLMs analyze this data to extract meaningful insights.
- Pattern Recognition: LLMs identify hidden relationships between drugs and diseases by processing natural language data, while knowledge graphs visualize these connections.
- Hypothesis Generation: The LLM knowledge graph drug purpose framework proposes potential drug-disease interactions, which researchers can test in laboratory settings.
- Drug Repurposing: This approach excels in identifying new therapeutic uses for existing drugs, reducing development timelines and costs.
Applications of LLM Knowledge Graph Drug Purpose in Healthcare
The implementation of LLM knowledge graph drug purpose has opened new avenues for innovation in healthcare:
- Drug Discovery: By analyzing vast biomedical datasets, this framework identifies promising drug candidates for diseases with unmet medical needs.
- Precision Medicine: The ability to tailor treatments to individual patients is enhanced through detailed insights into genetic and phenotypic data.
- Rare Disease Research: Knowledge graphs help connect sparse data points, enabling breakthroughs in understanding and treating rare conditions.
- Toxicity Prediction: Combining LLMs and knowledge graphs allows researchers to predict adverse drug reactions and optimize safety profiles.
Case Studies Demonstrating the Power of LLM Knowledge Graph Drug Purpose
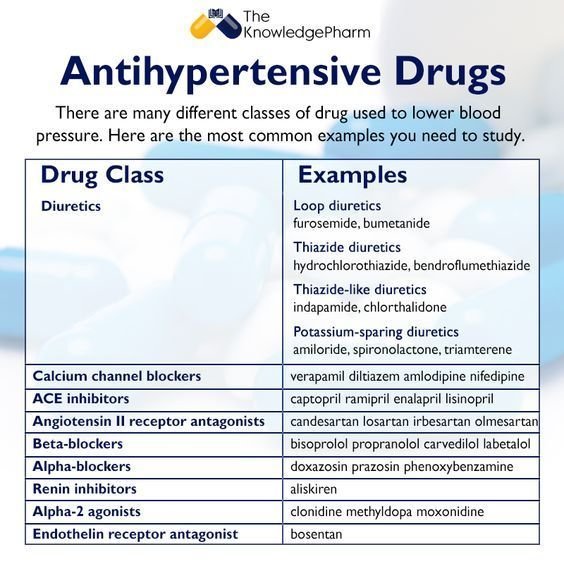
- COVID-19 Drug Repurposing: During the pandemic, researchers utilized LLM knowledge graph drug purpose to identify existing drugs with potential antiviral properties, significantly accelerating the response to the crisis.
- Cancer Therapy: Knowledge graphs enriched with LLM-driven insights have revealed novel biomarkers and drug targets for various cancers, paving the way for more effective treatments.
- Neurodegenerative Diseases: The framework has contributed to uncovering relationships between genetic mutations and neurological disorders, informing the development of targeted therapies.
Advantages of LLM Knowledge Graph Drug Purpose
The adoption of LLM knowledge graph drug purpose offers several benefits:
- Efficiency: Automating data analysis reduces the time required to identify drug candidates.
- Cost-Effectiveness: Drug repurposing minimizes the expense of traditional drug development.
- Scalability: The framework handles large datasets, making it suitable for global-scale research initiatives.
- Collaboration: Shared knowledge graphs foster interdisciplinary collaboration among researchers, clinicians, and data scientists.
Challenges and Limitations of LLM Knowledge Graph Drug Purpose
Despite its promise, LLM knowledge graph drug purpose faces several challenges:
- Data Quality: The accuracy of predictions depends on the quality and completeness of input data.
- Computational Resources: LLMs and knowledge graphs require significant processing power, which may be a barrier for smaller research institutions.
- Ethical Considerations: Ensuring patient data privacy and avoiding biases in AI algorithms are critical concerns.
- Validation: Experimental validation of AI-generated hypotheses remains essential to confirm their scientific validity.
Future Directions for LLM Knowledge Graph Drug Purpose
The evolution of LLM knowledge graph drug purpose is likely to include:
- Integration with Genomics: As genomic sequencing becomes more accessible, integrating this data with knowledge graphs will enhance precision medicine.
- AI Explainability: Developing transparent AI systems will improve trust and facilitate regulatory approval of AI-driven drug discoveries.
- Real-Time Analysis: Advancements in computing power will enable real-time updates to knowledge graphs, reflecting the latest research developments.
- Global Collaboration: International partnerships will create unified knowledge graphs, accelerating progress in tackling global health challenges.
Impact of LLM Knowledge Graph Drug Purpose on Pharmaceutical Research
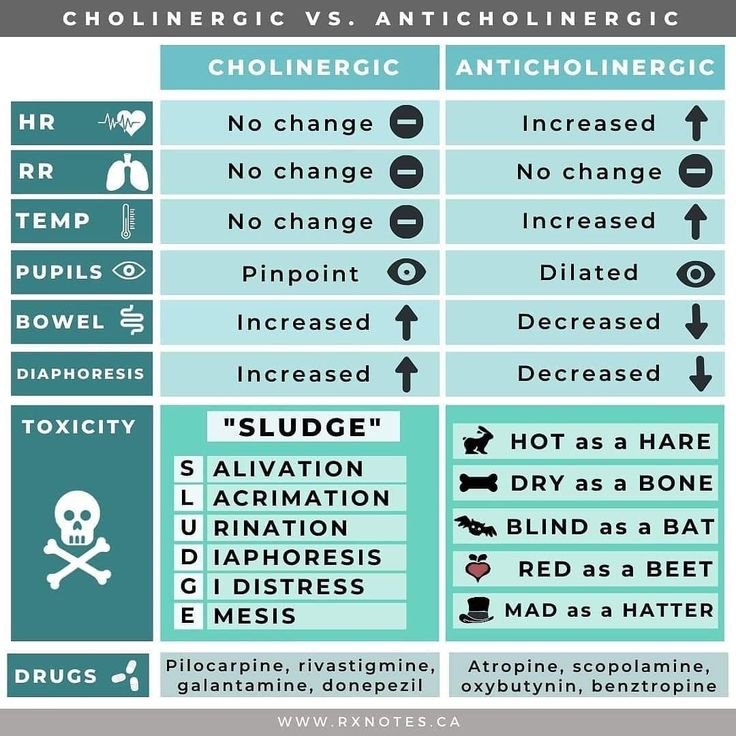
The LLM knowledge graph drug purpose approach is redefining the pharmaceutical landscape:
- Faster Development: AI-driven insights streamline the drug development pipeline, bringing treatments to market more quickly.
- Informed Decision-Making: Researchers gain a deeper understanding of complex biological systems, enabling more informed decisions.
- Innovative Therapies: The identification of unconventional drug targets fosters the creation of groundbreaking therapies.
Also read Did Trains Leave the Hurricane Path Earlu? Exploring Preparedness and Response
Conclusion
The intersection of AI, data science, and pharmacology through LLM knowledge graph drug purpose represents a transformative leap in medicine. By harnessing the combined strengths of LLMs and knowledge graphs, researchers can address some of the most pressing challenges in drug discovery and development. While challenges remain, the potential benefits of this approach make it a cornerstone of future healthcare innovations. As the field evolves, LLM knowledge graph drug purpose will undoubtedly play a pivotal role in shaping the future of medicine.